Unraveling variability in U.S. opinion on climate change
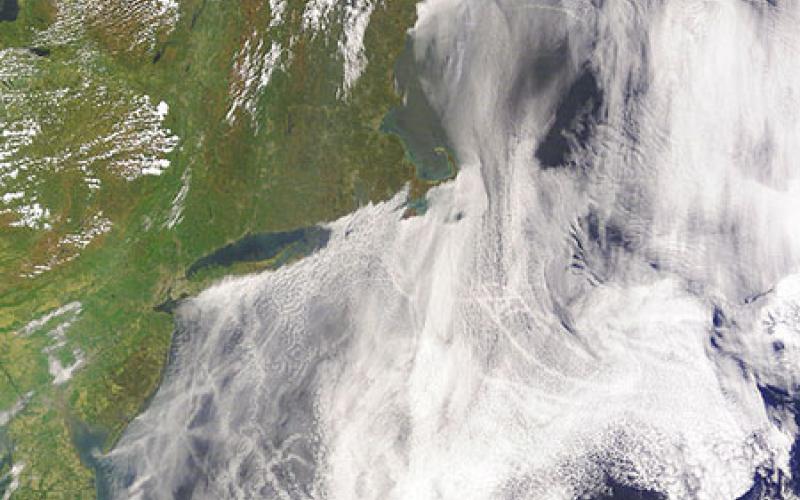
Unraveling variability in U.S. opinion on climate change
Although much research shows there are significant differences in public opinion on climate change beliefs, national scale statistics conceal this heterogeneity. Using an approach known as multilevel regression and poststratification, a team of researchers finds significant variability in opinion on important climate-related issues and behaviors at all levels of comparison.
Original Paper:
Howe, Peter D., Matto Mildenberger, Jennifer R. Marlon, and Anthony Leiserowitz. "Geographic variation in opinions on climate change at state and local scales in the USA." Nature Climate Change 5, no. 6 (2015): 596-603. DOI: http://dx.doi.org/10.1038/nclimate2583
While a large body of survey work and research indicates stark disagreement in public opinion on many topics, national-scale statistics obscure these differences. This results in a form of aggregation bias — or, a reduction in the variability we are able to see in data — that inaccurately portrays public opinion for most subgroups. From a policy perspective, this causes problems across all areas of social interest and particularly in implementing policy to address global climate change. Effective policy in this realm is thought to require action at all political scales, so being able to gauge public opinion and interest at finer geographic scales where action must occur is critical for success.
The approach is particularly advantageous for areas of the country with low population densities, outperforming other methods in terms of mean absolute error, a measure of statistical performance. In such areas traditional survey methods can often yield statistically insignificant or not generalizable results or are too expensive to conduct. The MRP approach includes two stages. In the first, a set of regression equations with dependent opinion variables and a variety of independent demographic, spatial, and time variables — as well as known proxies for harder to define aspects such as liberalism and carbon emission based behaviors — are estimated.
For this paper these regression equations are based on national public opinion survey data, from more than 13,000 respondents, collected by the Yale Project on Climate Change Communication (YPCCC). The resulting coefficients are then used to define and statistically weight more than 100,000 population types at the smallest and most diverse, county-level scale across the U.S. These population types and weights in the second stage are then used along with U.S. demographic data for each state, county, congressional district, and MSA to estimate public opinion at these small, otherwise impractical geospatial scales. The efficacy of these estimates are then tested internally against sample subsets and externally, comparing resulting error against disaggregation methods with success. External validation included testing their estimates against several independent state and MSA-level surveys, ensuring the robustness.
The paper is notable as much for its methodology as the specific results. As for the results, the authors present a national scale narrative many readers might expect: even in deeply red or blue states there exist bastions of dissension. Strong disagreements are found at all spatial scales and across all four opinion categories explored in the paper: climate change, risk perceptions, policy preferences, and climate change related behaviors. These differences represent as much as double-digit percentage variations from surrounding area, state, or national level statistics. This fact has strong policy implications; no single policy implementation may be optimal at any spatial scale. However, our best approximation in policy may be to design state or national scale policies with a substantial amount of flexibility at smaller political scales, firmly cushioned in carefully designed incentive structures.
More information the author's specific results, including interactive maps on environmental topics, is available at the YPCCC Web site.